Projects
Dynamic Tasking
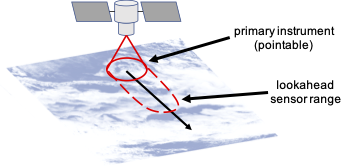
Graphic of lookahead sensor with spacecraft targeting primary sensor based on lookahead sensor data
Background
While recent advances in instrument and spacecraft technology have given rise to a new generation of miniaturized Earth observing instruments, the fundamental physics of remote sensing forces tradeoffs between aperture, swath, and resolution.
Typical NASA Earth Science missions have followed the “mow the lawn” paradigm of nadir pointed instruments blindly targeting based on orbit (static exceptions to this exist such as “instrument on over land”). In this paradigm large swath (high spatial coverage), high spatial resolution (in some cases vertical and horizontal), and high spectral resolution all drive costs upwards.
Mapping/”mow the lawn” techniques must acquire vast amounts of data to capture “by chance” rare events such as deep convective ice storms. Such techniques also acquire large amounts of cloudy data, which is of less utility for certain science applications (such as land surface).
The challenge is to sense intelligently to capture the most useful data within the constraints of the science instrument (swath, power/energy, agility) to maximize the science while keeping mission costs manageable.
Technology
Dynamic Tasking (DT) uses information from a lookahead sensor to identify targets for the primary sensor which can then be pointed or reconfigured to improve science yield. DT can be used with a single platform or across multiple platforms (aka Sensorweb). DT is applicable across a wide range of missions and we expect it to become commonplace across many if not most future Earth Science missions.
When overflying storm systems, a DT radar such as being developed in the SMICES Instrument Incubator Program effort [Swope et al. 2021] could be directed towards the deep convective core to increase measurement of these targets of interest. The radar configuration could be adjusted based on the type of target and type of science study to further improve return. And the DT instrument could track a single cell for an entire overflight to provide fine temporal scale evolution for 8-10 minutes overflight in low Earth orbit.
Alternatively, a forward looking sensor could detect areas of high gradient in temperature and moisture and direct lidar or radar to search for planetary boundary layer phenomena. When imaging the Earth’s surface, or even an atmospheric column (such as OCO-2 and OCO-3), clouds can interfere with measurement. In these cases, lookahead cloud detection (e.g. such as [Thompson et al. 2014] ) with DT could be used to target around clouds [Hasnain et al. 2021] to improve science return.
D. R. Thompson, R. O. Green, D. Keymeulen, S. Lundeen, Y. Mouradi, R. Castano, S. A. Chien, Rapid spectral cloud screening onboard aircraft and spacecraft. IEEE Transactions on Geoscience and Remote Sensing, 52(11), 2014, 6779 - 6792.
Impact
Preliminary analysis using simulated science datasets show the promise of Dynamic Tasking to increase capture of rare storm events by over 10x [Swope et al. 2021]
Preliminary analysis using actual MODIS cloud data [Hasnain et al. 2021] shows the promise of Dynamic Tasking to significantly increase the capture of non cloudy data (reduction from ⅔ of data cloudy to less than 10% cloudy.
Status
Early prototyping of algorithms for the SMICES Instrument Incubator Program effort began in 2019. Efforts to evaluate and generalize DT algorithms have followed.
Publications
Team
Steve ChienJason Swope
Alberto Candela Garza
Itai Zilberstein
Zaki Hasnain
James Mason
Joshua vander Hook
Sponsors
JPL FoundryEarth Science Technology Office, Instrument Incubation Program